Innolitics introduction 🔗
In our opinion, FDA is doing a great job keeping up with developments in AI/ML-enabled devices. One of the regulatory approaches they’re considering (and are likely to finalize in 2024), is the Predetermined Change Control Plan (PCCP). This is their draft guidance.
Innolitics specializes in Software as a Medical Device and AI/ML. See our other AI/ML resources and our portfolio for AI/ML projects we’ve completed. If you’d like help developing a PCCP for an AI/ML-enabled device, we’d be happy to help.
Preamble 🔗
This draft guidance document is being distributed for comment purposes only.
Document issued on April 3, 2023.
You should submit comments and suggestions regarding this draft document within 90 days of publication in the Federal Register of the notice announcing the availability of the draft guidance. Submit electronic comments to https://www.regulations.gov. Submit written comments to the Dockets Management Staff, Food and Drug Administration, 5630 Fishers Lane, Room 1061, (HFA-305), Rockville, MD 20852. Identify all comments with the docket number listed in the notice of availability that publishes in the Federal Register.
For questions about this document regarding CDRH-regulated devices, contact digitalhealth@fda.hhs.gov. For questions about this document regarding CBER-regulated devices, contact ocod@fda.hhs.gov. For questions about this document regarding CDER-regulated products, contact druginfo@fda.hhs.gov. For questions about this document regarding combination products, contact the Office of Combination Products at combination@fda.gov.
Contains non-binding guidance.
I. Introduction 🔗
FDA has a longstanding commitment to develop and apply innovative approaches to the regulation of medical device software and other digital health technologies to ensure their safety and effectiveness.1 As technology continues to advance all facets of healthcare, medical software incorporating artificial intelligence (AI), and specifically the subset of AI known as machine learning (ML) (henceforth referred to as machine learning-enabled device software functions or ML-DSFs), has become an important part of many medical devices. This draft guidance is intended to provide a forward-thinking approach to promote the development of safe and effective medical devices that use ML models trained by ML algorithms.
ML-enabled technologies have the potential to transform healthcare by deriving new and important insights from the vast amount of data generated during the delivery of healthcare every day. Medical device manufacturers2 are using ML technologies to innovate their products to better assist healthcare providers and improve patient care. Examples of ML applications in medicine include earlier disease detection and diagnosis, development of personalized diagnostics and therapeutics, and development of assistive functions to improve the use of devices with the goal of improving user and patient experience.
FDA recognizes that the development of ML-DSFs is an iterative process. This draft guidance proposes a least burdensome approach to support iterative improvement through modifications to an ML-DSF while continuing to provide a reasonable assurance of device safety and effectiveness. As such, this draft guidance demonstrates FDA’s broader commitment to developing innovative approaches to the regulation of device software functions as a whole. Specifically, this draft guidance provides recommendations on the information to be included in a Predetermined Change Control Plan (PCCP) provided in a marketing submission for an ML-DSF. This draft guidance recommends that a PCCP describe the planned ML-DSF modifications; the associated methodology to develop, implement, and validate3 those modifications; and an assessment of the impact of those modifications. The PCCP is reviewed as part of a marketing submission to ensure the continued safety and effectiveness of the device without necessitating additional marketing submissions for implementing each modification described in the PCCP.
In general, FDA’s guidance documents do not establish legally enforceable responsibilities. Instead, guidances describe the Agency’s current thinking on a topic and should be viewed only as recommendations, unless specific regulatory or statutory requirements are cited. The use of the word should in Agency guidances means that something is suggested or recommended, but not required.
II. Background 🔗
In April 2019, FDA published the Proposed Regulatory Framework for Modifications to Artificial Intelligence/Machine Learning (AI/ML)-Based Software as a Medical Device (SaMD) - Discussion Paper and Request for Feedback (“2019 discussion paper”).4 The 2019 discussion paper describes FDA’s foundation for a potential approach to premarket review for AI/ML-driven software The ideas delineated in the 2019 discussion paper leveraged practices from our current premarket programs and relied on the International Medical Device Regulators Forum’s risk categorization principles,5 the FDA’s benefit-risk framework,6 risk management principles described in the Software Modifications guidance,7 and the total product lifecycle approach envisioned in the Digital Health Software Precertification (Pre-Cert) Pilot Program.8
ML can allow software to learn through data, without being explicitly programmed, to perform a One of the greatest potential benefits of ML resides in the ability to improve ML model performance through iterative modifications, including by learning from real-world To support the iterative development of ML-DSFs, and as part of the proposed framework presented in the 2019 discussion paper, FDA described a “Predetermined Change Control Plan” that could be included in a marketing submission for a device that is or includes an ML-DSF (referred to interchangeably as an “ML-DSF” or a “device”).9 In this draft guidance, we provide recommendations on the marketing submission content for a PCCP, which generally includes: 1) a detailed description of the specific, planned device modifications; 2) the associated methodology to develop, validate, and implement those modifications in a manner that ensures the continued safety and effectiveness of the device across relevant patient populations, referred to as the “Modification Protocol”; and 3) an Impact Assessment to describe the assessment of the benefits and risks of the planned modifications and risk mitigations.
The 2019 discussion paper received a substantial amount of feedback from a wide array of General comments were received, as well as specific responses to 18 questions posed in the 2019 discussion10 Additionally, numerous articles in peer-reviewed journals discuss or reference the framework proposed in the 2019 discussion paper.11
FDA has also held a number of public meetings and workshops on AI/ML On February 25-26, 2020, FDA held a Public Workshop on the “Evolving Role of Artificial Intelligence in Radiological Imaging”12 to discuss emerging applications of AI in radiological imaging, including AI devices intended to automate the diagnostic radiology workflow as well as guide image At this workshop, the Agency worked with interested stakeholders, including patients, to identify both benefits and risks associated with the use of AI in radiological imaging, and discussed best practices for the validation of fully automated radiological imaging software and image acquisition devices.
On October 22, 2020, FDA held a Patient Engagement Advisory Committee meeting on “Artificial Intelligence and Machine Learning in Medical Devices”13 to further elicit input from a diverse group of patients on AI/ML The Committee provided recommendations on AI/ML-enabled medical devices and how to foster patient trust in them, considering the diverse populations in which they are and will be used.
On October 14, 2021, FDA held a Public Workshop on “Transparency of Artificial Intelligence/Machine Learning-enabled Medical Devices”14 for patients, caregivers, and The purpose of the workshop was to 1) identify unique considerations in achieving transparency for users of AI/ML-enabled medical devices and ways in which transparency might enhance the safety and effectiveness of these devices; and 2) gather input from various stakeholders on the types of information that would be helpful for manufacturers to include in the labeling and public facing information of AI/ML-enabled medical devices, as well as other potential mechanisms for information sharing.
FDA continues to receive an increasing number of marketing submissions and pre-submissions for devices leveraging ML technologies, and the Agency expects this to increase over Moreover, since the 2019 discussion paper’s publication, there has been strong interest in utilizing PCCPs for AI/ML-enabled medical devices.
In light of the public health need to facilitate innovation for ML-DSFs while providing appropriate oversight for them, on January 12, 2021, the CDRH Digital Health Center of Excellence issued FDA’s Artificial Intelligence/Machine Learning (AI/ML)-Based Software as a Medical Device Action Plan (“the Action Plan”).15 The Action Plan describes FDA’s strategy for addressing AI/ML-enabled medical devices in a holistic, collaborative, and multidisciplinary manner. An important pillar of the Action Plan is the further advancement of the tailored regulatory framework for ML-enabled medical devices that was proposed in the 2019 discussion paper.
Further, on October 4, 2022, the White House released a Blueprint for an AI Bill of Rights,16 which outlined five principles that should guide the design, use, and deployment of automated systems. These five principles discuss: safe and effective systems; algorithmic discrimination protections; data privacy; notice and explanation; and human alternatives, consideration, and fallback. This draft guidance is consistent with and promotes the principles described in the Blueprint for an AI Bill of Rights.
Additionally, section 3308 of the Food and Drug Omnibus Reform Act of 2022, Title III of Division FF of the Consolidated Appropriations Act, 2023, Pub. L. No. 117-328 (“FDORA”), enacted on December 29, 2022, added section 515C “Predetermined Change Control Plans for Devices” to the FD&C Act. Section 515C provides FDA with express authority to approve or clear PCCPs for devices requiring premarket approval or premarket notification. For example, section 515C provides that supplemental applications (section 515C(a)) and new premarket notifications (section 515C(b)) are not required for a change to a device that would otherwise require a premarket approval supplement or new premarket notification if the change is consistent with a PCCP previously approved or cleared by FDA. Section 515C also provides that FDA may require that a PCCP include labeling for safe and effective use of a device as such device changes pursuant to such plan, notification requirements if the device does not function as intended pursuant to such plan, and performance requirements for changes made under the plan.17 In this draft guidance, we provide recommendations on the marketing submission content for PCCPs, which are based on the statute and feedback obtained through our various interactions with stakeholders.
III. Scope 🔗
This draft guidance is applicable to ML-DSFs that the manufacturer intends to modify over time. This includes ML-DSFs for which modifications to the ML model are implemented automatically (i.e., for which the modifications are implemented automatically by software), as well as for ML-DSFs for which modifications to the ML model are implemented manually (i.e., involving steps that require human input, action, review, and/or decision-making, and therefore are not implemented automatically).
This draft guidance describes an approach that would often be least burdensome and would support the ability to modify an ML-DSF while continuing to provide a reasonable assurance of safety and effectiveness across relevant patient populations. Specifically, this draft guidance proposes recommendations on the information to be included in the PCCP in a marketing submission18 for a device that is or includes an ML-DSF. For the purposes of this guidance, the term “PCCP” refers to a plan that includes device modifications that would otherwise require a premarket approval supplement, De Novo submission, or a new premarket notification. A plan that contains only minor modifications that would not require a new submission is outside the scope of this guidance. For more information on whether a modification would require a new submission, see Deciding When to Submit a 510(k) for a Software Change to an Existing Device19 and Deciding When to Submit a 510(k) for a Change to an Existing Device20 or Modifications to Devices Subject to Premarket Approval (PMA) - The PMA Supplement Decision-Making Process21 guidances. Throughout this guidance, a PCCP that has been reviewed and established through a device marketing authorization is also referred to as an “authorized PCCP.” See Section IV. for more information on definitions used for the purposes of this guidance.
By including a PCCP in a marketing submission, manufacturers can proactively pre-specify and seek premarket authorization22 for intended modifications (and their method of implementation) to an ML-DSF without necessitating additional marketing submissions for each modification delineated and implemented in accordance with the PCCP. In other words, a PCCP, as part of a marketing submission, is intended to provide a means to implement modifications to an ML-DSF that generally would otherwise require additional marketing submissions prior to implementation.23
Modifications to an ML-DSF that could significantly affect,24 or that could affect,25 the safety or effectiveness of the device, unless those modifications are covered by a PCCP, require premarket authorization.26 Premarket authorization for an ML-DSF with a PCCP may be established through the 510(k) pathway (see section 515C(b) of the FD&C Act), PMA pathway (see section 515C(a) of the FD&C Act), or the De Novo pathway (see section 513(f)(2) of the FD&C Act).27 FDA considers the PCCP to be part of the technological characteristics of the device. For devices subject to 510(k) requirements, the determination of substantial equivalence includes, among other requirements, a comparison of the technological characteristics of the predicate device to the technological characteristics of the subject device.28 In making a determination of substantial equivalence where the predicate device was authorized with a PCCP, the subject device must be compared to the version of the predicate device cleared or approved prior to changes made under the PCCP.29
Generally, the recommendations in this guidance apply to the device constituent part of a combination product30 (such as drug-device and biologic-device combination products) when the device constituent part31 is or includes an ML-DSF. Early engagement with the appropriate CBER or CDER Divisions is recommended for drug-device and biologic-device combination products that include an ML-DSF.32 For more information, contact the FDA review division that will have the lead review for the combination product.
FDA encourages early engagement regarding a proposed PCCP with the FDA review division that will review the ML-DSF; in particular, early engagement could be especially helpful for certain ML-DSFs, including combination products and high-risk, life-sustaining, life-supporting, or implantable devices. FDA encourages manufacturers to leverage the Q-Submission process for obtaining FDA feedback on a proposed PCCP for an ML-DSF.33
This draft guidance is not intended to provide a complete description of what may be necessary to include in a marketing submission for an ML-DSF. It is also not intended to delineate the types of modifications the Agency would consider acceptable in a PCCP. However, some considerations on modification types are described, including examples of questions for consideration that manufacturers should address in a Modification Protocol (see Appendix A) and illustrations of different ML-DSF scenarios where a PCCP could be employed (see Appendix B). The FDA review division with purview over the device under review will determine the acceptability of a proposed PCCP. That is, the FDA review division will determine whether the scope of the modifications is appropriate for inclusion in a PCCP and what evidence and information are required to support proposed modifications in a marketing submission.34
IV. Definitions 🔗
This section defines certain terms as they are used for the purposes of this guidance.
A. Software Functions 🔗
Device Software Function (DSF): A software function that meets the device definition in section 201(h) of the FD&C Act.35ʼ36 As discussed in other FDA guidances, the term “function” is a distinct purpose of the product, which could be the intended use or a subset of the intended use of the product.37
Machine Learning-Enabled Device Software Function (ML-DSF): A device software function (as defined above) that implements an ML model trained with ML techniques.
B. Data Sets 🔗
Training Data: These data are used by the ML-DSF manufacturer in procedures and ML training algorithms to build an ML model, including to define model weights, connections, and components. Training the ML model happens after the exploratory phase of ML-DSF development. These data typically should be representative of the proposed intended use populations (e.g., with respect to race, ethnicity, disease severity, gender, age, etc.). The result is an operational ML-DSF.
Tuning Data: These data are typically used by the ML-DSF manufacturer to evaluate a small number of trained ML-DSFs in order to explore, for example different architectures or hyperparameters. The tuning phase is the last phase before ML-DSF testing and is often considered part of the training process. The ML community sometimes refers to the tuning data and phase with the word “validation.” However, we recommend that the word “validation” not be used when referring to data or operations related to training or tuning ML models intended for medical applications.38
Testing Data: These data are used to characterize the performance of the ML-DSF. The testing phase is expected to provide data to establish a reasonable assurance of safety and effectiveness before an ML-DSF is marketed. Testing data should be independent of data used for training and tuning and should be from multiple sites different from those that were used to generate training and tuning data.
C. Predetermined Change Control Plan 🔗
Predetermined Change Control Plan (PCCP): The documentation describing what modifications will be made to the ML-DSF and how the modifications will be assessed. The modifications described in the PCCP include device changes that would otherwise require a PMA supplement, De Novo submission, or new 510(k) notification. The PCCP includes a Description of Modifications, Modification Protocol, and Impact Assessment.
Authorized Predetermined Change Control Plan (Authorized PCCP): A PCCP that has been reviewed and established through a device marketing authorization. An authorized PCCP is a technological characteristic of the authorized device with which it was established.
Modification Protocol: The documentation describing the methods that will be followed when developing, validating, and implementing modifications delineated in the Description of Modifications section of the PCCP. The Modification Protocol includes the verification and validation activities (including pre-defined acceptance criteria) for those modifications and is intended to provide a step-by-step delineation of how the modifications proposed in the PCCP will be implemented while ensuring the device remains safe and effective.
Impact Assessment: The documentation of the assessment of the benefits and risks of implementing a proposed PCCP, as well as the plan for risk mitigation. It could be a separate section of a PCCP or incorporated into the Modification Protocol, whichever is least burdensome for a particular marketing submission.
V. Policy for Predetermined Change Control Plans 🔗
Software development is an iterative process, and FDA appreciates that manufacturers of device software functions strive to continually improve and update their devices. Manufacturers should evaluate the impact of modifications to their devices and must submit a marketing submission when device modifications affect the intended use of the device or could significantly affect the safety or effectiveness of the device.39
An authorized PCCP specifies planned modifications that, if not included in a PCCP, could otherwise require a new marketing submission pursuant to 21 CFR 807.81(a)(3) and 21 CFR 814.39(a), and in accordance with Deciding When to Submit a 510(k) for a Software Change to an Existing Device40 and Deciding When to Submit a 510(k) for a Change to an Existing Device41 or Modifications to Devices Subject to Premarket Approval (PMA) - The PMA Supplement Decision-Making Process42 guidances (hereafter referred to as the “device modifications guidances”). An authorized PCCP includes the “range of FDA-authorized specifications” for the characteristics and performance of the planned modifications to the device (as part of the detailed Description of Modifications section included in the PCCP), along with the associated verification and validation testing and acceptance criteria to assure the device remains safe and effective across relevant patient populations (as part of the Modification Protocol section included in the PCCP), and documentation of the assessment of the benefits and risks of implementing a proposed PCCP (which could be a separate Impact Assessment section of a PCCP or incorporated into the Modification Protocol). Because modifications made to an ML-DSF in accordance with an authorized PCCP were reviewed and authorized through the marketing submission containing the PCCP, the modifications can be implemented to the ML-DSF without triggering the need for a new marketing submission under 21 CFR 807.81(a)(3) and 21 CFR 814.39(a), and in accordance with the device modifications guidances.
Deviations from the authorized PCCP reviewed in the marketing submission could significantly affect the safety or effectiveness of the device. For example, when implementing a modification that is a deviation from the PCCP, the device’s clinical functionality or performance specifications could be compromised. Deviations from the PCCP include instances where the PCCP is not followed or cannot be followed (e.g., issues related to the Modification Protocol, such as data management, re-training, or performance failure). Accordingly, modifications made to an ML-DSF that are not specified in, or implemented in accordance with, the authorized PCCP (i.e., the manufacturer deviates from the authorized PCCP when implementing the modification) likely require a new marketing submission.43ʼ44 In such a circumstance, continued distribution of the ML-DSF without submitting a new marketing submission would constitute adulteration and misbranding under sections 501(f)(1)(B) and 502(o) of the FD&C Act, respectively. Adulteration and misbranding are prohibited acts under section 301 of the FD&C Act, and where appropriate, FDA may take legal or regulatory action against violations of prohibited acts, including, without limitation, seizure or injunction.
A. Components of a PCCP 🔗
A PCCP should consist of a detailed Description of Modifications, a Modification Protocol, and an Impact Assessment (see Sections VI. – VIII.) because these components are intended to provide FDA with the information needed for our review of the proposed modifications. The detailed Description of Modifications should outline the modifications that will be made to the ML-DSF, and the Modification Protocol should describe the verification and validation activities (including pre-defined acceptance criteria) that will support those modifications. The Impact Assessment helps to tie the Description of Modifications to the Modification Protocol in that the Impact Assessment identifies the benefits and risks introduced by the specified, planned modifications and how the verification and validation activities of the Modification Protocol will continue to assure the safety and effectiveness of the device. The detailed Description of Modifications, Modification Protocol, and Impact Assessment are all components of a PCCP and should exist in tandem.
B. Establishing a PCCP 🔗
Premarket authorization for an ML-DSF with a PCCP must be established through the 510(k) pathway, De Novo pathway, or PMA pathway, as appropriate, as a PCCP must be reviewed and established as part of a marketing authorization for a device prior to a manufacturer implementing any modifications under that PCCP.45 For 510(k) submissions, in making a determination of substantial equivalence where the predicate device was authorized with a PCCP, the subject device must be compared to the version of the predicate device cleared or approved prior to changes made under the PCCP.46
For a manufacturer who would like to establish a new PCCP for a previously authorized device with a PCCP, the marketing submission must include the appropriate marketing submission requirements47 and the proposed PCCP for the device (see Section V.E.).48 FDA intends to focus its review on the aspects of the device that are most significantly modified.49 For example, if the device has been relatively unchanged since FDA’s prior authorization and a new PCCP is proposed, FDA would focus its review on the proposed PCCP.
An authorized PCCP is a technological characteristic of the authorized device with which it was established. If the authorized device is significantly modified other than as specified in the authorized PCCP, a new marketing submission is required for the significantly modified device.50 In that case, for the original authorized PCCP to apply to the significantly modified device, the PCCP will need to be reviewed and established as part of such marketing submission.
FDA encourages manufacturers to leverage the Q-Submission process for obtaining FDA feedback on a proposed PCCP prior to submitting a marketing submission. For more information on the Q-Submission process, refer to the Requests for Feedback and Meetings for Medical Device Submissions: The Q-Submission Program guidance.51 While manufacturers are encouraged to discuss their plans through a Pre-Submission, PCCPs are not authorized in Pre- Submissions.
C. Identifying a PCCP in a Marketing Submission 🔗
The PCCP should be included as a standalone section within the marketing submission. Additionally, it should be prominently included and discussed in the cover letter and included in the marketing submission’s table of contents as “Predetermined Change Control Plan.” The PCCP should be discussed in the marketing submission as part of the device description, labeling, and relevant sections used for determining substantial equivalence or reasonable assurance of safety and effectiveness. Any information pertaining to the PCCP content included outside of the PCCP section should be referenced within the PCCP section.
Device labeling must comply with applicable statutes and regulations,52 which includes adequate directions for use.53 FDA may require that a change control plan include labeling required for safe and effective use of the device as such device changes pursuant to such plan.54 For ML- DSFs with an authorized PCCP, the labeling should explain that the device incorporates machine learning and has a PCCP so that users are aware that the device may require the user to perform software updates, and that such software updates may modify the device’s performance, inputs, or use. Information on the ML-DSF and its PCCP in the labeling is important in order for a user to use the device safely and for the purposes for which it is intended. In particular, this information may be necessary for a user to understand changes in the device and to continue to use the device safely and effectively across relevant populations as the device changes pursuant to the PCCP.
The PCCP should be described in the 510(k) summary,55ʼ56 De Novo decision summary,57 or PMA summary of safety and effectiveness document (SSED) and approval order.58 Details of the PCCP should be included in sufficient detail in the public-facing documents to support transparency to users of FDA’s determination of substantial equivalence or reasonable assurance of safety and effectiveness for the device and its range of FDA-authorized specifications.
D. Utilizing an Authorized PCCP to Implement Device Modifications 🔗
Once a PCCP has been reviewed and established through a marketing submission, the PCCP is considered part of the marketing authorization. In general, a PCCP should be evaluated within the existing risk management framework of the device and implemented in accordance with the manufacturer’s quality system.
Figure 1 depicts the process for implementing a modification to a device with an authorized PCCP. Manufacturers should first consider whether the modification is or is not consistent with the authorized PCCP; a modification is considered consistent with the authorized PCCP when the modification has been specified in the Description of Modifications included in the PCCP and has been implemented in accordance with the Modification Protocol. If the modification is consistent with the authorized PCCP, a new marketing submission is not necessary, the modification can be implemented in accordance with the Modification Protocol, and the manufacturer should document that modification and the analysis in accordance with the manufacturer’s quality system.59
As described previously, an authorized PCCP specifies planned modifications that, if not included in a PCCP, would otherwise require a new marketing submission pursuant to 21 CFR 807.81(a)(3) and 21 CFR 814.39(a), and in accordance with the device modifications guidances. Therefore, if the modification is not consistent with the authorized PCCP – including if the specific modification is not included in the authorized PCCP or if the modification is included in the authorized PCCP but is not implemented in accordance with the methods and specifications described in the Modification Protocol – the manufacturer should then proceed to evaluate the modification in accordance with applicable laws and regulations and the device modifications guidances, if applicable, and proceed accordingly. If, after review of applicable laws and regulations, a new marketing submission is required,60 then the manufacturer must submit the appropriate marketing submission before the modified device is marketed. The appropriate marketing submission could request authorization for 1) a device modification effected through a change to the authorized PCCP61 (see Section V.E.); or 2) a device modification not implemented through a PCCP; or 3) both. In each of these cases, a marketing submission for the device modification must include the appropriate marketing submission requirements62 for the device. As noted in Section V.B., an authorized PCCP is a technological characteristic of its authorized device with which it was established. Therefore, for the original authorized PCCP to apply to the modified device, the manufacturer must include the proposed PCCP in the marketing submission for the device modification;63 the PCCP is reviewed and established as part of the review of the modified device.
See Appendix B for example scenarios for implementing modifications to a device with an authorized PCCP. Manufacturers may contact the appropriate FDA review division (e.g., through a Pre-Submission)64 for a discussion about the proposed modification and whether it may be considered consistent with the current, authorized PCCP.
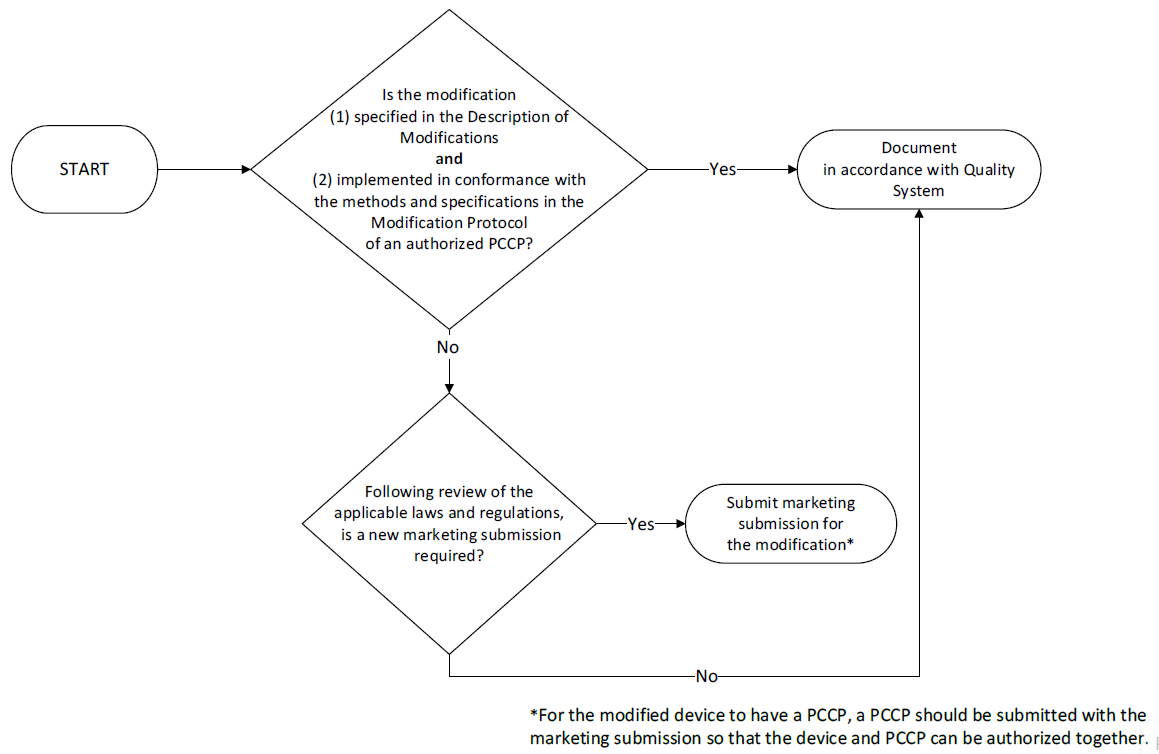
E. Modifying a PCCP for an Authorized Device 🔗
Because the modifications described in the PCCP include device changes that would otherwise require a PMA supplement, De Novo submission, or new 510(k) premarket notification, at this time, FDA believes that modifications to an authorized PCCP will generally constitute changes to the ML-DSF that require a new marketing submission for the device, which will include the modified PCCP.65 In other words, FDA expects that the modified PCCP will need to be reviewed and established as part of the premarket review of the modified device because a modification to the PCCP will generally significantly affect the safety or effectiveness of the device.66 In those cases where modifications to the PCCP are the only significant modifications introduced since FDA’s prior authorization for the device and its PCCP, FDA intends to focus its review on the proposed PCCP. For a manufacturer who would like to modify a PCCP for a previously authorized device with a PCCP, the marketing submission must include the appropriate marketing submission requirements for the device and the proposed PCCP.67
VI. Description of Modifications 🔗
As introduced above, a description of each planned modification to an ML-DSF should be included in the Description of Modifications section of a PCCP. The detailed description should describe changes to the device characteristics and performance resulting from implementation of the modifications. To ensure an efficient review, FDA recommends that a PCCP include only a limited number of modifications that are specific, and that can be verified and validated.
A. Goals of the Description of Modifications Section 🔗
A dedicated Description of Modifications section in a PCCP identifies the specific, planned modifications to the ML-DSF that the manufacturer intends to implement. The Description of Modifications draws a “range of FDA-authorized specifications” around the initial characteristics and performance of the device that, following the agreed upon verification and validation described in the Modification Protocol, can be implemented without a new marketing submission. Upon FDA review of a PCCP, it is possible that FDA may determine that a Modification Protocol supports some but not all modifications identified in a PCCP; in such cases, only those modifications that are appropriate in the FDA’s findings of substantial equivalence or reasonable assurance of safety and effectiveness would be included in the authorized PCCP.
B. Content of the Description of Modifications Section 🔗
The Description of Modifications should enumerate the list of individual proposed device modifications discussed in the PCCP, as well as the specific rationale for the change to each part of the ML-DSF that is planned to be modified. In some situations, a Description of Modifications will consist of multiple modifications. It may be helpful to reference the labeling changes that are associated with each modification in the Description of Modifications section (such labeling changes should be included in a Modification Protocol, as described in Section VII.B.).
FDA recommends that a PCCP include modifications that are specific, and that can be verified and validated. Modifications should also be presented at a level of detail that permits understanding of the specific modifications that will be made to the ML-DSF. Each modification should be linked to a specific performance evaluation activity within the Modification Protocol (for an example, see Table 1 in Section VII.C.).
The Description of Modifications should clearly state if the planned modifications are proposed to be implemented automatically (i.e., whether the modifications are implemented automatically by software) or whether modifications are implemented manually (i.e., involving steps that require human input, action, review, and/or decision-making, and therefore are not implemented automatically). Understanding that this is an evolving area, FDA is proposing to consider PCCPs for ML-DSFs where modifications are implemented automatically to the extent the Agency can properly review them for substantial equivalence to the predicate or a reasonable assurance of safety and effectiveness. The Agency recognizes that this subset of ML-DSFs has an additional degree of complexity; as with all ML-DSFs, FDA will consider the benefit-risk assessment, and the Agency’s current experience applying this policy when reviewing PCCPs containing automatically implemented modifications.
The Description of Modifications should also clearly specify if the proposed modifications will be implemented in a uniform manner across all devices on the market (sometimes referred to as homogenous or global changes, or global adaptations) or implemented differently on different devices on the market based on, for example, the unique characteristics of a specific clinical site or individual patients (sometimes referred to as heterogenous or local changes, or local adaptations). For local adaptations, the Description of Modifications should include describing what local factors or conditions warrant a local change.
C. Types of Modifications 🔗
Modifications that are appropriate for a PCCP include those that are intended to maintain or improve the safety or effectiveness of the device. Modifications proposed within the Description of Modifications should be able to be verified and validated within the existing quality system of the device. As such, not all modifications may be appropriate for inclusion within a PCCP. Types of modifications that may be acceptable within a PCCP include:
- modifications related to quantitative measures of ML-DSF performance specifications;
- modifications related to device inputs to the ML-DSF; and
- limited modifications related to the device’s use and performance (e.g., for use within a specific subpopulation).
Examples of modifications related to quantitative measures of ML-DSF performance specifications include improvements to analytical and clinical performance resulting from re- training the ML model based on new data within the intended use population from the same type and range of input signal.
Modifications related to device inputs to the ML-DSF may involve expanding the algorithm to include new sources of the same signal type (e.g., different makes, models, or versions of a data acquisition system) or limited modifications related to new types of inputs.
Modifications related to the device’s use and performance could include authorization of a device for a specific subset of a population within the originally indicated population based on re-training on a larger data set for that subpopulation that was not previously available.
All modifications included in a PCCP must maintain the device within the device’s intended use.68 At this time, FDA expects that modifications included in a PCCP should also maintain the device within the device’s indications for use.69 As with modifications to the intended use, FDA believes modifications to the indications for use would not allow the device to remain safe and effective.70
Recognizing there is a spectrum of risk for devices, for the purposes of reviewing a PCCP, FDA intends to, among other considerations, take into account the benefit-risk profile of the specific device that is the subject of the PCCP, the specific modifications being proposed, and its experience applying this policy across different device types. See Appendix B for examples of modifications.
VII. Modification Protocol 🔗
The Modification Protocol includes the documentation describing the methods that will be followed when developing, validating, and implementing modifications delineated in the Description of Modifications section of the PCCP. The Modification Protocol includes the verification and validation activities (including pre-defined acceptance criteria) for those modifications and is intended to provide a step-by-step delineation of how the modifications proposed in the PCCP will be implemented while assuring the device remains safe and effective. Documentation of modifications verified and validated per the Modification Protocol must be compliant with the quality system (QS) regulation.71 The QS regulation requires manufacturers of finished medical devices to review and approve modifications to device design and production (21 CFR 820.30 and 820.70) and document changes and approvals in the device master record (21 CFR 820.181).
A. Goals of the Modification Protocol Section 🔗
Whereas the Description of Modifications outlines the planned modifications to an ML-DSF, the Modification Protocol describes the methods that will be followed when developing, validating, and implementing those modifications, to ensure the device remains safe and effective. The methods described in the Modification Protocol should be consistent with and support the modifications outlined in the Description of Modifications.
The goals of the Modification Protocol are to:
- Identify the methods and data used to develop, validate, and implement all proposed modifications;
- Identify the test methods, data, statistical analyses, and specified acceptance criteria for all proposed modifications;
- Ensure that the information that would otherwise be generated and submitted to the Agency (i.e., if the modifications were implemented on a device that did not have an authorized PCCP) will be generated by the manufacturer for each modification and maintained consistent with recordkeeping requirements72 and in accordance with the manufacturer’s QS;
- Ensure that the risks that have been identified in the Impact Assessment as related to modifications detailed in the Description of Modifications (including the update process and communication to users) will be mitigated; and
- Be least burdensome for the manufacturer to develop and for FDA to review. This includes being traceable and specific to the modifications detailed in the Description of Modifications section and sufficiently comprehensive to support specific modifications.
This draft guidance identifies four primary components of a Modification Protocol, described below, that outline a manufacturer’s 1) data management practices, 2) re-training practices, 3) performance evaluation protocols, and 4) update procedures, including communication and transparency to users and real-world monitoring plans, for each modification in a PCCP. In FDA’s experience, these four components generally provide FDA with the information needed to evaluate the PCCP. For a particular marketing submission, additional components of a Modification Protocol may be important to provide a reasonable assurance of safety and effectiveness.
In some cases, the same methods in each component of the Modification Protocol may support all modifications in a PCCP for a device. In other cases, the same methods in each component may not be adequate for every modification in a PCCP. It is important to note, however, that for each planned modification provided in the Description of Modifications, FDA recommends that each of the four primary components of a Modification Protocol be addressed. Additionally, the Modification Protocol should include a description of how its proposed methods are similar to or different from methods used elsewhere in the marketing submission. For example, if the validation methods in the Modification Protocol represent a subset of the original testing for the device, or if the acceptance criteria for the validation are different, manufacturers should describe these differences and provide a justification. The justification for a different methodology may include references to other marketing submissions where the methodology was used for similar modifications.
As noted above in Section V.E., manufacturers should follow their risk management processes to develop a Modification Protocol that considers each modification. Upon FDA review of a PCCP, FDA may determine that the Modification Protocol supports some but not all proposed modifications identified in the PCCP. In such cases, only those modifications that were supportable by the proposed Modification Protocol would be appropriate for inclusion in the PCCP and would become part of the authorized PCCP. Those modifications that were not supportable by the proposed Modification Protocol, or that FDA considered inappropriate for other reasons (e.g., the modification changes the device’s indications for use), would be removed from the PCCP.
B. Content of the Modification Protocol Section 🔗
As part of their Modification Protocol, manufacturers should outline the methods for each component described below. Example elements of each of the four Modification Protocol components are provided in Appendix A.
(1) Data management practices 🔗
What they are: ML-DSF training and testing typically utilize data that include the inputs (e.g., medical images) that will be used by the device and often utilize a label or ‘reference standard’ that is determined through a reference standard determination and/or an annotation process. Training data and testing data are sequestered to prevent overfitting and misquotes of test performance. The training and testing methods aim to identify and eliminate bias in the data (which may be inherent from historical datasets) and to improve the robustness and resilience of these algorithms to withstand changing clinical inputs and conditions. To support modifications to an ML-DSF that may need training and/or testing, it is anticipated that new data (i.e., data that were not used to develop the initial ML-DSF) will be collected. The data management practices in a Modification Protocol should outline how those new data will be collected, annotated, curated, stored, retained,73 controlled, and used by the manufacturer for each modification. The data management practices in a Modification Protocol should also clarify the relationship between the Modification Protocol data and the data used to train and test the initial and subsequent versions of the ML-DSF. It should also describe the control methods employed to curb the potential for data or performance information leaking into the development process during modification development or assessment.
Why they are recommended: This information allows FDA to understand the manufacturer’s data management practices that will be used to support each modification to an ML-DSF, including 1) how the manufacturer plans to obtain and use training and testing data that are complete and representative of the proposed intended use populations (e.g., with respect to race, ethnicity, disease severity, gender, age, etc.74); 2) whether identifiable subpopulations will be adequately represented, including intersectional groups, and separated into training and testing sets to minimize ML model bias; 3) how training and testing data will be sequestered to prevent overfitting and misquotes of test performance; 4) how older data will be complemented or replaced by newer data so that the performance is representative of the current patient population and standard of care; 5) whether the reference standard represents the best available process for determining the ground truth; and 6) how the data management practices may reduce the potential to produce discriminatory outcomes. A clear explanation of data management practices also provides assurance to FDA that modifications to the ML-DSF are based on data that are representative of the device indications for use. This includes information regarding products that will be used to generate data as inputs for the ML-DSF, patient populations in which the device will be used, and clinical scenarios where the device will be used.
What manufacturers should include in a submission: Examples of the types of information manufacturers should provide in a Modification Protocol describing their data management practices are provided in Appendix A. In general, this information should describe: how data will be collected, including clinical study protocols with inclusion/exclusion criteria; information on how data will be processed, stored, and retained;75 the process that will be followed to determine the reference standard; the quality assurance process related to the data; the data sequestration strategies that will be followed during data collection to separate the data into training and testing sets; and the protocols in place to prevent access during the training and tuning process to data intended for performance testing.
(2) Re-training practices 🔗
What they are: ML software generally involves multiple processing steps from the point the ML- DSF receives the input data to the point it provides an output. The re-training practices component of a Modification Protocol should identify the processing steps that are subject to change for each modification and the methods that will be used by the manufacturer to implement modifications to the ML-DSF. In addition, if re-training involves ML architecture modifications (e.g., in a neural network, modifications to training hyperparameters or the number of nodes, layers, etc.), the re-training practices component of a Modification Protocol should also describe the rationale or the justification for each specific architecture modification.
Why they are recommended: Information on the manufacturer’s re-training practices allows FDA to understand how the proposed modifications will be achieved through re-training, to determine if modifications are implemented following appropriate, well-defined practices,76 and to determine if the performance evaluation and update procedures (discussed below) support the modifications. Information on the manufacturer’s re-training practices is typically provided in the “device description” of a marketing submission for the majority of ML-DSFs that FDA reviews. The specifics of what should be included in this component of the Modification Protocol will depend on the type of modification and specific device.
What manufacturers should include in a submission: Examples of the types of information manufacturers should provide in a Modification Protocol describing their re-training practices are provided in Appendix A. In general, this information should identify the objective of the re-training process, provide a description of the ML model, identify the device components that may be modified, outline the practices that will be followed (e.g., data sequestration strategies during re-training), and identify any triggers for re-training (e.g., when new data reaches a certain size or when a drift in data is observed over time).
(3) Performance evaluation 🔗
What they are: The FDA may require that performance requirements for changes made under the plan be provided in a PCCP.77 Performance evaluation methods should describe the processes that will be followed to validate that the modified ML-DSF will meet the specifications identified as part of a specific modification, in addition to maintaining the specifications that are not part of the modification but may be impacted by the modification. Performance evaluation should include the plans for verification and validation of the entire device following ML-DSF modifications for each individual modification and in aggregate for all implemented modifications. This includes, but is not limited to, ML model testing protocols comparing the newly modified device to both the original device (the version of the device without any modifications implemented) and the last modified version of the device. For example, for device software functions that drive hardware functionality, performance evaluation should include not only the device software functions, but also the effect of the modifications on hardware functionality. The content of this section in a Modification Protocol should provide details on the study design, performance metrics, pre-defined acceptance criteria, and statistical tests for each planned modification. More comprehensive testing can potentially support a broader set of proposed modifications.
Why they are recommended: Information regarding the manufacturer’s performance evaluation methods allow FDA to confirm that appropriate study designs, including performance metrics and statistical tests, will be used to evaluate the effect of modifications on overall device performance. Performance evaluation of the device is essential to ensure that specified acceptance criteria for all proposed modifications will continue to be met over the range of FDA- authorized specifications.
What manufacturers should include in a submission: Examples of the types of information manufacturers should provide in a Modification Protocol describing their performance evaluation are provided in Appendix A. In general, this information should describe how performance evaluation will be triggered; how sequestered test data representative of the clinical population and intended use will be applied for testing; what performance metrics will be computed; and what statistical analysis plans will be employed to test hypotheses relevant to performance objectives for each modification. In addition, the Modification Protocol should affirmatively state that if there is a failure in performance evaluation for a specific modification, the failure(s) will be recorded, and the modification will not be implemented.
(4) Update procedures 🔗
What they are: Data management practices, re-training practices, and performance evaluation described above largely relate to making and testing modifications to the ML-DSF. Once these meet the performance objectives, manufacturers will need to update the ML-DSF to implement the modifications and communicate information to users about the modifications that is needed to safely use the device. The update procedures in a Modification Protocol should describe how manufacturers will update their devices to implement the modifications, provide appropriate transparency to users, and, if appropriate, updated user training about the modifications and perform real-world monitoring, including notification requirements if the device does not function as intended pursuant to the authorized PCCP.78 The PCCP should include a description of any labeling changes that will result from the implementation of the modifications. The available labeling must include adequate directions for use and reflect information about the current version(s) of the ML-DSF available to the user, including information regarding site- specific modifications.79 The labeling should not reflect information on modifications to the ML- DSF that have not been implemented in the available version, because it could cause confusion and would be misleading. If such information is included in the labeling, the ML-DSF could be deemed misbranded.80
Why they are recommended: Information on the manufacturer’s update procedures allows FDA to understand 1) how risks from implementing modifications may be mitigated by the update process; 2) how communication regarding the device updates will be provided to users (e.g., so that updates in device output results will be correctly interpreted by users); 3) how the device operation will remain reliable after the update; and 4) how all stakeholders will be kept up-to- date about device functionality and performance. In addition, it is important for FDA to understand how potential risks associated with the update process, itself, may be mitigated.
What manufacturers should include in a submission: Examples of the types of information manufacturers should provide in a Modification Protocol describing their update procedures are provided in Appendix A. In general, this information should include 1) confirmation that the verification and validation plans for the modified version of the device are the same as those that have been performed for the version of the device prior to the implementation of the modifications, or identification of any differences between the two plans; 2) a description of how software updates will be implemented; 3) a description of how legacy users will be affected by the software update (if applicable); and 4) a description of how modifications will be communicated to the users, including transparency on any differences in performance, differences in performance testing methods, and/or known issues that were addressed in the update (e.g., whether there is an improvement in performance in a subpopulation of patients). Communication of performance changes should be consistent with performance evaluation described in the Modification Protocol.
C. Traceability Between the Description of Modifications Section and the Modification Protocol Section 🔗
The PCCP should clearly delineate which parts of the Modification Protocol are applicable to each modification within the Description of Modifications. For a PCCP with multiple modifications, this may be accomplished through a traceability table; a sample traceability table is provided below in Table 1. This sample traceability table provides an example of how a manufacturer can depict the traceability between the Description of Modifications and Modification Protocol, as well as how to provide clear references to where within the PCCP this information is located in a marketing submission.
Table 1. Example of Description of Modifications to Modification Protocol Traceability Table
Table 1: A traceability table can help to identify where each method supporting each modification may be found in the marketing submission.
Modification Protocol Component |
Modification Protocol Component | Modification Protocol Component | Modification Protocol Component | |
---|---|---|---|---|
Modification |
Data management practices | Re-training practices | Performance evaluation | Update procedures |
Modification #1 |
Method A (see Section X.A) | Method D (see Section X.D) | Method G (see Section X.G) | Method J (see Section X.J) |
Modification #2 |
Method A (see Section X.A) | Method E (see Section X.E) | Method H (see Section X.H) | Method J (see Section X.J) |
Modification #3 |
Method B (see Section X.B) | Method F (see Section X.F) | Method I (see Section X.I) | Method J (see Section X.J) |
VIII. Impact Assessment 🔗
An Impact Assessment, in the context of a PCCP, is the documentation of the assessment of the benefits and risks of implementing a PCCP for an ML-DSF, as well as the mitigations of those risks. The manufacturer’s existing quality system should be used as the framework in which to conduct an Impact Assessment for the modifications set forth in the PCCP.
Documentation for an Impact Assessment provided to the Agency in a marketing submission containing a PCCP should 1) compare the version of the device with each modification implemented to the version of the device without any modifications implemented, 2) discuss the benefits and risks, including risks of social harm, of each individual modification, and 3) discuss how the activities proposed within the Modification Protocol continue to reasonably ensure the safety and effectiveness of the device. The Impact Assessment documentation in a marketing submission should also discuss 4) how the implementation of one modification impacts the implementation of another, and 5) the collective impact of implementing all modifications. FDA believes it is important to address these elements in an Impact Assessment in order to demonstrate that the combination of the proposed modifications is unlikely to introduce additional, unmitigated risks, and that the safety and effectiveness of the device under review is maintained as modifications are implemented.
Impact Assessment documentation in a marketing submission should discuss how the individual modifications included in the PCCP impact not only the ML-DSF, but also how they impact the overall functionality of the device, including how they impact other device software functions, as well as device hardware. Additionally, if the ML-DSF is a device function of a multiple function device product, we recommend considering FDA’s guidance Multiple Function Device Products: Policy and Considerations81 to determine if any information should be included in the Impact Assessment documentation in a marketing submission (and the marketing submission overall) so that FDA may assess the impact of the “other function(s)” on the safety or effectiveness of the ML-DSF and modifications to the ML-DSF.
The Agency acknowledges that for some devices, it may be least burdensome to include the content of the Impact Assessment within the Modification Protocol rather than as a separate section within the PCCP.
Appendix A: Example Elements of Modification Protocol Components for ML-DSFs 🔗
In general, a Modification Protocol that is included as part of a PCCP in a marketing submission should include four components that outline a manufacturer’s 1) data management practices, 2) re-training practices, 3) performance evaluation protocols, and 4) update procedures, for each modification in the Description of Modifications for the ML-DSF. However, manufacturers may include other or additional components if they believe that their proposed protocols do not fit into any of these four components. Examples of questions for consideration and the types of information manufacturers should provide in these components of a Modification Protocol are provided below.
Note that this is a developing area, and as FDA gains experience, these example questions may change. The items below are also not an exhaustive list of topics that a manufacturer is expected to cover, and all questions may not apply to all marketing submissions. Likewise, the Agency may request additional Modification Protocol components or information to be included in a PCCP for some device types so that the Agency can review the PCCP as part of the marketing submission.82 Some sections of a Modification Protocol may be more or less detailed depending on the complexity and risks of each modification in the PCCP.
(1) Data Management 🔗
Different data can be collected and used for training and testing ML model updates. For collection of new training and testing data, the Modification Protocol should include how the data will be used (e.g., for ML model development or testing), and how the data management supports these uses.
a. Collection protocols
- For each modification, what are the inclusion/exclusion criteria for data collection, and how are they linked to the intended use population?
- What is the intended distribution of your data set along covariates describing the patient population (e.g., sex, age, race, height, weight, disease conditions) and data acquisition conditions (e.g., sites, data acquisition devices/methods, imaging and reconstruction protocols)? Is this distribution representative of the intended use population, including intersectional groups?
- Will the data be collected prospectively or retrospectively? Will the data set include consecutive cases within a given date range? Otherwise, if random sampling is planned, what method or technique will be used and how will it account for bias and randomness?
- Are there any plans for enrichment or stratified sampling to include specific patient subgroups (e.g., sex, age, race)?
- What is the number and geographical distribution of data collection sites?
- What are the measures to mitigate potential unwanted bias in learning or performance estimation, for example, due to issues related to new training or testing data, respectively?
- What are the strategies and measures to understand and mitigate potential biases in the data, such as those due to historical inequalities to medical treatment access by different populations?
- What are the strategies to ensure data sets remain relevant over time with respect to changes in, for example, data acquisition technologies or protocols, clinical practice, patient populations, and disease conditions?
- Are data collection, storage, retention, and use protocols in compliance with regulations for human subject protections and requirements for clinical investigations (e.g., pursuant to 21 CFR Part 812, 45 CFR Part 46, 21 CFR Part 50, and 21 CFR Part 506, as applicable)?
- For ML-DSFs that use input data from dedicated acquisition systems (e.g., software device functions in a patient monitor that uses connected sensors), is the data acquired with the systems and settings with which the ML-DSF will be used? For device software functions that use input data from different acquisition systems (e.g., interoperable medical devices), does the data acquired meet the input specifications of the ML-DSF?
b. Assurance of Data Quality
- What techniques will be employed to fortify data consistency and completeness?
- What are the strategies used to promote data authenticity, transparency, and integrity?
- How will potentially missing data elements within a case/record be handled?
- Are there criteria for including/excluding cases/records based on data quality (in addition to inclusion/exclusion criteria listed in 1.a.1. above), and if so, what are the criteria and rationale?
- If data might be excluded as a result of the quality assurance process, what methods are planned to minimize the impact on the generalizability of training and accuracy of testing?
- Are there strategies to trace a data issue to an individual record? What are the strategies to identify and investigate data issues?
- Will data that are obsolete or no longer needed be removed?
- What controls are in place to prevent and identify unauthorized access or manipulation of the training and testing data sets? For example, what controls are in place to prevent malicious addition or deletion of data for the purpose of adversarial machine learning?
- Is there an automated process to ensure data quality?
c. Reference standard determination
For the purposes of this guidance, the reference standard is the best available truth standard to define the true condition for each patient/case/record. The reference standard may be used in device training, device testing, or both.
- What is the justification for the method for the determination of the reference standard?
- If the reference standard is based on evaluations from clinicians, what was the grading protocol used (e.g., what are the total number of clinicians who participated and their qualifications; what data are these clinicians provided with; and how are the clinicians’ evaluations collected/adjudicated for determining the reference standard)?
- What is the strategy for addressing cases where results obtained using a reference standard may be equivocal or missing?
- What is the uncertainty inherent in your reference standard?
- Will any of the methods for determining the reference standard be automated? Are there differences between the reference standards used for training versus testing?
- Are there differences between the reference standard used to support the initial ML-DSF marketing authorization and the reference standard being applied to update the ML model?
d. Sequestration of test data sets
For the purposes of this guidance, sequestration of test data sets means that manufacturers do not have access to the test data set for the purpose of ML-DSF development.
- What strategies will be employed at the outset of data collection to shield the test data set from the ML-DSF development?
- What are the specific procedures to be followed so that the test data set remains sequestered during re-training?
- If test data are planned to be used multiple times for performance evaluation, what measures are in place to prevent unwanted bias from being introduced through ML model manufacturers learning substantial information about the test data set and results?
(2) Re-Training 🔗
a. Re-training objectives and focus
- How are the modifications presented in the Description of Modifications in the PCCP related to the planned re-training methods?
- Which parts of the ML-DSF are planned to be modified (e.g., transfer learning, data pre-processing, data augmentation, only a certain set of coefficients, ML architecture and hyper-parameters, loss functions, optimization methods and criteria, types of ML model inputs and outputs), and what are the details of the planned modifications to the ML-DSF design? What is the specific rationale for the change to each part that is planned to be modified?
- For each part of the ML-DSF that will be modified, is ML model re-training needed to achieve the modifications specified in the PCCP?
- If re-training applies to only certain parts of the ML-DSF, what are the plans to ensure that other functions or software components are not affected?
b. Re-training implementation:
- What are the triggers for re-training (e.g., when new data reaches a certain size, when a drift is observed, periodically in time)?
- What strategies will be employed to identify and limit overfitting?
- Are there risks related to ML model bias introduced by re-training a modified ML model, and if so, what are planned mitigations?
(3) Performance Evaluation 🔗
a. Triggers to initiate performance evaluation
- What are the triggers for initiating performance evaluation of a re-trained ML model or modified ML-DSF (e.g., re-training shows a certain performance level on the training or validation data, test data reaches a certain size, periodically in time)?
- How frequently is this expected to occur?
b. Assessment metrics and elements
- How is the Data Management Plan in (1) above applied to produce the testing data for performance evaluation that are different from any training or tuning data?
- What metrics will be computed to understand device performance?
- How do these metrics demonstrate that the modified device can be safely used?
- How will the metrics provide a comprehensive assessment of device performance and patient safety?
- What corner cases (i.e., cases outside the norm) or known challenging scenarios will be evaluated?
c. Statistical analysis plans
- What is the plan for evaluating equivalent or improved performance with respect to previously validated versions, including the original version, of the ML-DSF?
- What are the high-risk subpopulations and subgroups (e.g., sex, gender differences, acquisition protocols) that need to be evaluated?
- How will this evaluation be used to support labeling specifications?
- How will you test that performance in one area (e.g., sensitivity) does not result in degrading performance in another (e.g., specificity)?
- How will the sample size be determined?
- Is the primary analysis based on the intention-to-diagnose population (no study subjects will be excluded) or the per-protocol population (subjects with protocol violations will be excluded)?
- How is variability in the reference standard accounted for (e.g., in the case of reader variability when clinical interpretation is used)? When the reference standard may be imperfect (e.g., sometimes includes a diagnostic error), are errors made by the imperfect reference standard conditionally independent of errors made by the ML-DSF, or are they positively correlated?
- How will missing data be addressed in analysis?
d. Performance targets
- What are the acceptance criteria?
- What clinical considerations were used to develop the acceptance criteria?
- How will the acceptance criteria support that the modification will be successfully implemented?
e. Additional testing needs
- Is database testing sufficient to address the risks associated with the proposed modification (e.g., does the user need to interact with the device to evaluate the performance or address a clinical risk or, for software that is part of a hardware device,83 how is the effect of the modification on hardware functionality evaluated)?
- How may clinical usability need to be addressed for a modification?
(4) Update Procedures 🔗
a. Software verification and validation
- Does the proposed modification necessitate a different software verification and validation plan from that used for the version of the device without any modifications implemented?
- What type of testing will be performed? Will the modified device be validated to function in an integrated environment?
- If the device includes other device functions in addition to the ML-DSF, how will the impact of modifications to the ML-DSF on the other device functions be evaluated?
- If the device includes “other functions” in addition to the ML-DSF, how will the “other functions’” impact on the safety or effectiveness of the modified ML-DSF be evaluated?
b. When and how updates will be implemented
- How will the decision be made on when to perform an update? What is the expected timeline for implementing the modifications? Is there a set frequency of updates?
- When and how will an update be implemented (e.g., automatically when the device is not being used, manually by users or hospital technicians, or both manually and automatically)? What is the basis on which the mechanism of implementation is dependent?
- For ML-DSF updates to reusable medical equipment, how will the device operation, including function of critical safety features (e.g., medical device alarms), be verified following the update?
- Will updates be made globally (i.e., the same update applied to all devices in the field) or locally (e.g., the devices may be modified for a patient/provider/care unit/hospital)?
- What cybersecurity protocols84 will be applied during updates?
c. Communication and transparency to users
- How will the PCCP be described in the public summary document and/or labeling?
- How will updates be communicated to users, including, but not limited to, in updated labeling (e.g., release notes)?
- What information about modifications to the device (e.g., performance) will be communicated to the user?
- How will version information be presented to the user when reviewing device outputs?
- Will users have the option to review labeling before implementing an update?
- How will any known biases or other performance issues with the potential to result in individual or social harms be disclosed, including, but not limited to, in labeling?
- How will any changes in performance related to known issues or sources of bias be communicated to the user, including, but not limited to, in labeling?
- What information about the population and methods for validation will be provided?
- If patient data from previous device use is available and can be rerun on an updated ML model, will this activity be performed for the available data and will those updated results be available to patients and users? Is there a plan to communicate if patient results before and after an update would provide clinically meaningful differences?
d. Device monitoring plan
- How will adverse events be tracked for different updates?
- Is there a plan to monitor real-world device performance (beyond general controls) and, if not, why is it not necessary?
- How will changes in performance in patient subpopulations be identified?
- What will be the response to the identification of previously unknown risks or previously unrecognized high-risk patient subpopulations?
- What is the strategy to respond to unexpected performance deficiencies or other hazards, or to higher levels of adverse events, as compared with previous iterations of the device?
- How will errors in diagnosis (i.e., misdiagnosis), attributable or partially attributable to the device that do not meet the criteria for an adverse event, be tracked?
Appendix B: Example ML-DSF Scenarios Employing PCCPs 🔗
The examples in this appendix illustrate different ML-DSF scenarios where a PCCP could be employed. Due to the complexity of ML-DSFs, all examples are hypothetical and do not reflect any specific authorized device.
Each example begins with a brief description of an authorized device, its intended use, and one summary of a modification from the Description of Modifications in its authorized PCCP (in the examples, denoted as “Brief Overview of Pre-Specified Modification”). Please note that the provided summaries of the devices and modifications in this appendix are not intended to reflect the complete content or detail expected in a Description of Modifications section in a PCCP. Rather, proposed modifications should be described in much greater detail in a PCCP, consistent with the recommendations provided throughout this guidance. The post-authorization modification scenarios are described to illustrate how the PCCP would be implemented. A distinction is drawn between post-authorization modifications that 1) would be acceptable for the authorized device with a PCCP and could be implemented without a new marketing submission or 2) would not be acceptable for the authorized device with a PCCP and likely requires a new marketing submission85 before the device could be introduced into interstate commerce.
Due to the complexity of ML-DSFs, it is not practical to describe all relevant considerations, or a complete PCCP, for the limited examples presented below. Therefore, while these examples highlight important concepts that could inform the development and utility of a PCCP, the PCCP will be specific to the circumstances of a particular ML-DSF, based on factors including a scientifically valid assessment of benefits and risks.
FDA recommends that the PCCP strategy be discussed with the appropriate FDA review division through the Q-Submission program86 prior to submitting a marketing submission containing a PCCP. As part of a marketing submission, the manufacturer should provide a PCCP, consisting of a Description of Modifications (Section VI), a Modification Protocol (Section VII), and an Impact Assessment (Section VIII).
(1) Patient Monitor Software 🔗
Background:
The device is an ML-DSF intended for use in high-acuity healthcare environments (e.g., an intensive care unit). The software obtains physiological signals (e.g., electrocardiogram, blood pressure, pulse oximetry) from a primary patient monitor. The physiological signals are processed and analyzed by an ML model to detect patterns that occur at the onset of physiologic instability. When physiologic instability is detected, an audible alarm signal is generated to indicate that prompt clinical action is needed to prevent potential harm to the patient. The ML- enabled medical device was authorized with a PCCP.
Brief Overview of Pre-Specified Modification:
The manufacturer would like to re-train the ML model with more data to reduce the false alarm rate while maintaining or increasing sensitivity to the onset of physiologic instability. The baseline sensitivity is y%. The manufacturer would like to demonstrate a significant improvement in the false-alarm rate while the sensitivity remains within a pre-specified non-inferiority margin of z% when compared with the original device, i.e., the version of the device without any modifications.87
Post-Authorization Modification Scenarios:
Modification Scenario 1: Modification related to quantitative measures of device performance, as specified in the PCCP, and implemented in accordance with the PCCP
In accordance with the Modification Protocol, data were collected and used to re-train the ML model. The modified ML model was tested per the methods specified in the Modification Protocol. The results demonstrated that the false alarm rate was significantly reduced while the mean sensitivity estimate was statistically within the proposed non-inferiority margin of the baseline sensitivity y%. Labeling was updated in accordance with the modified ML-DSF performance, and communication was provided to the device users. Because the device modification was specified in the PCCP, and it was implemented in conformance with the PCCP, the device modification would not require a new marketing submission. The manufacturer should document the modification that was specified in the PCCP in accordance with their quality system.
Modification Scenario 2: Modification beyond quantitative measures of device performance, which was not specified in the PCCP
In accordance with the Modification Protocol, the manufacturer re-trained their ML model using additional data to improve the sensitivity. Analytical validation demonstrated that the revised ML model has the same false alarm rate and sensitivity as the previous version. However, the manufacturer also noticed that the modified ML model maintained the same sensitivity and can now also predict physiologic instability in advance of its onset, which the previous version of the ML model could not do. The manufacturer would like to update the device’s indications for use to reflect this additional performance claim to predict physiologic instability in advance of its onset, which was not previously included in the PCCP. The methods used for analysis, performance, and statistics were not specified in the PCCP for predicting a future state. Because this modification that was not included in the PCCP could significantly affect the safety or effectiveness of the device, a new marketing submission would be required.
(2) Skin Lesion Software 🔗
Background:
The device is an ML-DSF that analyzes images of skin lesions by identifying and characterizing its features (e.g., color, quantification of area change over time) to aid in diagnosis. It was validated with a specific camera and is intended to be used by a primary healthcare provider. The device was authorized with a PCCP.
Brief Overview of Pre-Specified Modification:
The manufacturer would like to extend the ML-DSF for use on additional general-purpose computing platforms, including smartphones and tablets. The general-purpose computing platform must include a two-dimensional camera that meets the minimum specifications defined in the PCCP. The updated device must achieve a minimum performance defined in the Modification Protocol using a specified methodology.
Post-Authorization Modification Scenarios:
Modification Scenario 1: Modification in input, as specified in the PCCP and implemented in accordance with the PCCP
The manufacturer’s analytical validation demonstrated the ML-DSF can be deployed on two additional smartphones that have image acquisition specifications that meet the minimum specifications provided in the PCCP. The analytical performance using the new image acquisition systems was found to be statistically equivalent to the baseline performance, as specified in the Modification Protocol. Labeling was updated to reflect the new ML-DSF compatibility with the additional smartphones, which may increase access of the ML-DSF in the healthcare community. Communication updates on device compatibility were also provided. Because the device modification was specified in the PCCP, and it was implemented in conformance with the PCCP, the device modification would not require a new marketing submission. The manufacturer should document the modification that was specified in the PCCP in accordance with their quality system.
Modification Scenario 2: Modification in input that was not specified in the PCCP
The manufacturer would like to deploy a modified ML model that uses images captured by a thermographic camera; however, the new camera technology was not specified in the PCCP. Because this modification that was not included in the PCCP could significantly affect the safety or effectiveness of the device, a new marketing submission would be required.
Modification Scenario 3: Modification related to the device’s use and performance, which was not specified in the PCCP
The manufacturer would like to distribute a new version of the ML-DSF that is patient- facing. The ML-DSF would provide an analysis of the physiological characteristics of skin lesions, as it does currently, and direct patients to follow-up with a dermatologist based on the preliminary analysis of the malignancy of the skin lesion. The modification introduces many new, unconsidered risks that were not yet mitigated in the current PCCP, given that the modified ML-DSF will be patient-facing. Because this modification that was not included in the PCCP could significantly affect the safety or effectiveness of the device, a new marketing submission would be required.
(3) Ventilator Settings Software 🔗
Background:
The device is an ML-DSF intended for use in the healthcare or home-use setting. The ML-DSF recommends the ideal ventilation parameters based on input data interpretation, which can then be programmed into the ventilator by a healthcare provider. The manufacturer proposes modifications to the ML-DSF to improve performance within the original indications. The device was authorized with a PCCP.
Brief Overview of Pre-Specified Modification:
The manufacturer would like to re-train the ML model to optimize site-specific performance for a specific subset of patients with a particular condition, for whom sufficient data were not previously available. Specifically, the manufacturer would like to modify the ML model to improve its ability to optimize ventilator settings for minute volume and tidal volume to reduce the variability to ±x% within the specified range to improve treatment outcomes for that subset of patients at different sites.
Post-Authorization Modification Scenarios:
Modification Scenario 1: Modification related to the device’s use and performance in a subset of the patient population, which was specified in the PCCP and implemented in accordance with the PCCP
The manufacturer re-trained and re-validated the ML model on newly acquired data in a subpopulation of patients with a particular disorder. As evidenced by additional clinical performance data collected and analyzed per the Modification Protocol, the re-training on new data improved the reliability and precision of ventilator setting recommendations, showing improvements and specializations to improve site-specific ventilator operation. The updated recommendations were validated against patient outcomes and adverse events that may occur due to ventilator setting inaccuracies following the methods in the Modification Protocol. The adverse event rates and outcomes acceptance criteria were established in the Modification Protocol, and as such, were used to validate the updated ML model. The ML-DSF was updated to implement the re-trained ML model and the labeling was updated for clarity to inform users how the updated ML model accounts for local experience and prevalence. The implementation of this modification was done only at applicable sites. Because this device modification was specified in the PCCP, and it was implemented in conformance with the PCCP, the device modification would not require a new marketing submission. The manufacturer should document the modification that was specified in the PCCP in accordance with their quality system.
Modification Scenario 2: Modification related to device’s use and performance in a subset of the patient population, which was specified in the PCCP, but was not implemented in conformance with the PCCP
The manufacturer re-trained and re-validated the ML model on newly acquired data, but was unable to fulfill the protocol because the manufacturer had to implement a reference standard that was different from the one described in the Modification Protocol. Even though the modification was specified in the PCCP, it was not implemented in conformance with the PCCP. Because this modification that was not implemented in conformance with the PCCP could significantly affect the safety or effectiveness of the device, a new marketing submission would be required.
(4) Image Acquisition Assistance Device 🔗
Background:
The ML-DSF is integrated into an imaging system and is intended to assist healthcare providers during acquisition of ultrasound images of the shoulder region in adult and pediatric populations by highlighting portions of the image where it detects a potential abnormality in real time. The ML-DSF interfaces with the device acquisition system, analyzes its output using an ML model, provides real-time alerts to the operator if an abnormality is detected, and automatically adjusts parameters in the device acquisition system during image acquisition to optimize the imaging. The device does not provide a diagnosis. The ML-enabled medical device was authorized with a PCCP.
Brief Overview of Pre-Specified Modification:
The manufacturer would like to re-train their ML model to further optimize the accuracy of the abnormality detection. The PCCP pre-specifies that both the sensitivity and specificity will be shown to be significantly superior for abnormality identification during the shoulder exam.
Post-Authorization Modification Scenario:
Modification Scenario 1: Modification related to quantitative measures of device performance, as specified in the PCCP, and implemented in accordance with the PCCP
In accordance with the Modification Protocol, imaging data were collected and used to re-train the ML model. The modified ML model was tested according to a specified test protocol in the Modification Protocol. The results demonstrated that the sensitivity and specificity for abnormality identification met statistical superiority pre-specifications. Labeling was updated in accordance with the modified device performance, and communication was provided to the device users. Because the device modification was specified in the PCCP, and because it was implemented in conformance with the PCCP, the device modification would not require a new marketing submission. The manufacturer should document the modification that was specified in the PCCP in accordance with their quality system.
Modification Scenario 2: Modification related to the device’s use and performance that was not specified in the PCCP
The manufacturer used new images to re-train the ML model and would like to update their labeling to reflect improved performance in the same shoulder region in a subset of the pediatric patient population identified in the device’s indications for use. However, the modification was not specified in the PCCP. Because this modification that was not included in the PCCP could significantly affect the safety or effectiveness of the device, a new marketing submission would be required.
(5) Feeding Tube Placement Radiograph Analysis Software 🔗
Background:
The device is an ML-DSF that analyzes chest radiographs from hospitalized patients to evaluate feeding tube placement. The ML-DSF reorders the radiologist’s review queue so that radiographs identified as having a higher likelihood of feeding tube misplacement are placed higher in the queue. The device was authorized with a PCCP.
Brief Overview of Pre-Specified Modification:
The manufacturer would like to improve ML model performance by increasing sensitivity to misplaced feeding tubes by re-training on new data. The baseline sensitivity is x%.
Additionally, the manufacturer would like to modify the device so that it would notify nursing staff to check on the patient, in parallel with prioritization in the review queue. This modification requires achieving a sensitivity of z%.
Post-Authorization Modification Scenarios:
Modification Scenario 1: Modification related to quantitative measures of device performance, and modification related to device’s use and performance, which were both specified in the PCCP and implemented in accordance with the PCCP
The manufacturer re-trained and re-validated the ML model on newly acquired data as described in the Modification Protocol, which significantly improved the ML-DSF sensitivity from x% to z% to detect incorrect feeding tube placements. The improved sensitivity achieved the required sensitivity (z%) statistically to enable the nursing staff notification function, and that notification function was enabled. Labeling of the device was changed in accordance with the PCCP. Because the device modification was specified in the PCCP, and it was implemented in conformance with the PCCP, the device modification would not require a new marketing submission. The manufacturer should document the modification that was specified in the PCCP in accordance with their quality system.
Modification Scenario 2: Modification related to device’s use and performance that was not specified in the PCCP
The manufacturer used the same database of images to re-train the ML model to identify pneumonia on chest radiographs. The pneumonia identification function was found to have the same sensitivity and specificity as the feeding tube ML model. The manufacturer would like to employ the new pneumonia identification function feature alongside the feeding tube placement ML model in radiograph triage. The modification was not specified in the PCCP. Because this modification that was not included in the PCCP could significantly affect the safety or effectiveness of the device, a new marketing submission would be required.
Footnotes 🔗
-
FDA regulates software that meets the definition of a device, which is defined in section 201(h)(1) of the Federal Food, Drug, and Cosmetic Act (FD&C Act) as “an instrument, apparatus, implement, machine, contrivance, implant, in vitro reagent, or other similar or related article, including any component, part, or accessory, which is – recognized in the official National Formulary, or the United States Pharmacopoeia, or any supplement to them, intended for use in the diagnosis of disease or other conditions, or in the cure, mitigation, treatment, or prevention of disease, in man or other animals, or intended to affect the structure or any function of the body of man or other animals, and which does not achieve its primary intended purposes through chemical action within or on the body of man or other animals and which is not dependent upon being metabolized for the achievement of its primary intended purposes. The term ‘device’ does not include software functions excluded pursuant to section 520(o)” of the FD&C Act. ↩
-
For the purposes of this guidance, “manufacturer” is used in accordance with the definitions of manufacturer in 21 CFR Parts 803, 806, 807, and 820 and as described in FDA’s guidance “Policy for Device Software Functions and Mobile Medical Applications” (DSF-MMA) available at https://www.fda.gov/regulatory-information/search-fda- guidance-documents/policy-device-software-functions-and-mobile-medical-applications. ↩
-
For the purposes of this guidance, the term “validation” is being used as it is defined in 21 CFR 820.3(z), which states “[v]alidation means confirmation by examination and provision of objective evidence that the particular requirements for a specific intended use can be consistently fulfilled.” See Section IV. for more information on definitions used for the purposes of this guidance. ↩
-
Available at https://www.fda.gov/medical-devices/software-medical-device-samd/artificial-intelligence-andmachine-learning-software-medical-device. ↩
-
See FDA’s website on “Global Approach to Software as a Medical Device” available at
https://www.fda.gov/medical-devices/software-medical-device-samd/global-approach-software-medical-device and
IMDRF’s SaMD Risk Categorization Framework available at
http://www.imdrf.org/docs/imdrf/final/technical/imdrf-tech-140918-samd-framework-risk-categorization141013.pdf. ↩ -
See FDA’s guidance “Factors to Consider When Making Benefit-Risk Determinations in Medical Device
Premarket Approval and De Novo Classifications” available at https://www.fda.gov/regulatory-information/searchfda-guidance-documents/factors-consider-when-making-benefit-risk-determinations-medical-device-premarketapproval-and-de and FDA’s guidance “Benefit-Risk Factors to Consider When Determining Substantial Equivalence in Premarket Notifications (510(k)) with Different Technological Characteristics” available at https://www.fda.gov/regulatory-information/search-fda-guidance-documents/benefit-risk-factors-consider-whendetermining-substantial-equivalence-premarket-notifications-510k. ↩ -
See FDA’s guidance “Deciding When to Submit a 510(k) for a Software Change to an Existing Device” available at https://www.fda.gov/regulatory-information/search-fda-guidance-documents/deciding-when-submit-510ksoftware-change-existing-device. ↩
-
See FDA’s website on “Digital Health Software Precertification (Pre-Cert) Program” available at
https://www.fda.gov/medical-devices/digital-health-center-excellence/digital-health-software-precertification-precert-program. ↩ -
For the purposes of this guidance, the terms “ML-DSF” and “device” are used interchangeably. Additionally, reference to an “ML-DSF” is referring to a software function that meets the definition of device, as defined in section 201(h) of the FD&C Act. See Section IV. for details on definitions. ↩
-
For more information, see the 2019 discussion paper’s public docket available at
https://www.regulations.gov/document/FDA-2019-N-1185-0001. ↩ -
For example, Gerke S et al., “The need for a system view to regulate artificial intelligence/machine learning-based software as medical device,” NPJ Digit Med 3, 53 (2020); Harvey et al., “How the FDA Regulates AI,” Academic Radiology 27, 58-61 (2020); and Subbaswamy et al., “From development to deployment: dataset shift, causality, and shift-stable models in health AI,” Biostatistics 21, 345-352 (2020). ↩
-
Available at https://www.fda.gov/medical-devices/workshops-conferences-medical-devices/public-workshopevolving-role-artificial-intelligence-radiological-imaging-02252020-02262020. ↩
-
Available at https://www.fda.gov/advisory-committees/advisory-committee-calendar/october-22-2020-patientengagement-advisory-committee-meeting-announcement-10222020-10222020. ↩
-
Available at https://www.fda.gov/medical-devices/workshops-conferences-medical-devices/virtual-publicworkshop-transparency-artificial-intelligencemachine-learning-enabled-medical-devices. ↩
-
Available at https://www.fda.gov/medical-devices/software-medical-device-samd/artificial-intelligence-andmachine-learning-software-medical-device. ↩
-
Available at https://www.whitehouse.gov/ostp/ai-bill-of-rights/. ↩
-
Sections 515C(a)(3) and (b)(3) of the FD&C Act. ↩
-
For purposes of this guidance, unless otherwise stated, the term “marketing submission” includes premarket notification (510(k)) submission, De Novo Classification request, and Premarket Approval (PMA) application. ↩
-
Available at https://www.fda.gov/regulatory-information/search-fda-guidance-documents/deciding-when-submit- 510k-software-change-existing-device. ↩
-
Available at https://www.fda.gov/regulatory-information/search-fda-guidance-documents/deciding-when-submit- 510k-change-existing-device. ↩
-
Available at https://www.fda.gov/regulatory-information/search-fda-guidance-documents/modifications-devices- subject-premarket-approval-pma-pma-supplement-decision-making-process. ↩
-
For the purposes of this guidance, the term “authorization” includes clearance of a 510(k) submission, grant of a De Novo Classification request, or approval of a PMA application. ↩
-
21 CFR 807.81(a)(3) and 21 CFR 814.39(a). ↩
-
21 CFR 807.81(a)(3). ↩
-
21 CFR 814.39(a). ↩
-
In accordance with 21 CFR 807.81(a)(3), a 510(k) is required for significant changes or modifications to a device and include 1) those that “could significantly affect the safety or effectiveness of the device, e.g., a significant change or modification in design, material, chemical composition, energy source, or manufacturing process” or include 2) “a major change or modification in the intended use of the device.” In accordance with 21 CFR 814.39(a), a PMA supplement is required for “change[s] affecting the safety or effectiveness of the device” unless an exception applies (see 21 CFR 814.39). For simplicity, in this guidance, we state “significantly” affect the safety or effectiveness of the device” for when a marketing submission may be required, “significant” modifications, and similar phrasings, aligning with 21 CFR 807.81(a)(3). However, for devices subject to PMA requirements, the broader requirement pursuant to 21 CFR 814.39(a) of a “change affecting the safety or effectiveness” applies. ↩
-
The De Novo classification process allows FDA to classify a device into class I or II when general controls or general controls and special controls provide a reasonable assurance of safety and effectiveness, but for which there is no legally marketed predicate. The De Novo pathway, therefore, allows FDA to develop special controls that provide a reasonable assurance of the safety and effectiveness. At this time, FDA expects that if it authorizes an ML- DSF with a PCCP via the De Novo pathway, the Agency would develop appropriate special controls, which may include specific requirements for a PCCP. ↩
-
See section 513(i) of the FD&C Act. ↩
-
See section 515C(c) of the FD&C Act. ↩
-
21 CFR 3.2(e). ↩
-
CFR 4.2. ↩
-
See FDA’s guidance “Requesting FDA Feedback on Combination Products” available at https://www.fda.gov/regulatory-information/search-fda-guidance-documents/requesting-fda-feedback-combination- products. ↩
-
See FDA’s guidance “Requests for Feedback and Meetings for Medical Device Submissions: The Q-Submission Program” available at https://www.fda.gov/regulatory-information/search-fda-guidance-documents/requests- feedback-and-meetings-medical-device-submissions-q-submission-program. ↩
-
21 CFR 807.81(a)(3) and 21 CFR 814.39(a). ↩
-
See footnote 1. ↩
-
Device software functions may include Software as a Medical Device (SaMD) and Software in a Medical Device (SiMD). See FDA’s website on “Software as a Medical Device (SaMD),” available at https://www.fda.gov/medicaldevices/digital-health-center-excellence/software-medical-device-samd. ↩
-
See FDA’s guidance “Multiple Function Device Products: Policy and Considerations” available at
https://www.fda.gov/regulatory-information/search-fda-guidance-documents/multiple-function-device-productspolicy-and-considerations. ↩ -
The way the term “validation” is used in the ML community to refer to training or tuning data sets is not
consistent with the broad understanding of the word “validation,” defined in 21 CFR 820.3(z) as “confirmation by examination and provision of objective evidence that the particular requirements for a specific intended use can be consistently fulfilled.” ↩ -
21 CFR 807.81(a)(3) and 21 CFR 814.39(a) ↩
-
Available at https://www.fda.gov/regulatory-information/search-fda-guidance-documents/deciding-when-submit- 510k-software-change-existing-device. ↩
-
Available at https://www.fda.gov/regulatory-information/search-fda-guidance-documents/deciding-when-submit- 510k-change-existing-device. ↩
-
Available at https://www.fda.gov/regulatory-information/search-fda-guidance-documents/modifications-devices- subject-premarket-approval-pma-pma-supplement-decision-making-process. ↩
-
21 CFR 807.81(a)(3) and 21 CFR 814.39(a). ↩
-
See Section V.D. below for further details on implementing device modifications that may or may not require a new marketing submission in accordance with 21 CFR 807.81(a)(3) and 21 CFR 814.39(a). ↩
-
See sections 513(f)(2) and 515C of the FD&C Act. ↩
-
See section 515C(c) of the FD&C Act. ↩
-
See, e.g., 21 CFR 807.87, 21 CFR 860.220, or 21 CFR 814.20. ↩
-
E.g., through a Traditional 510(k) for a device that has already been authorized, or a PMA supplement, such as a panel-track supplement or 180-day supplement. ↩
-
Note that “focus of the review” is not intended to imply a review of the PCCP only; rather, the focus on the PCCP is as a significant change to the device that could affect the safety or effectiveness of the device. ↩
-
21 CFR 807.81(a)(3) and 21 CFR 814.39(a). ↩
-
Available at https://www.fda.gov/regulatory-information/search-fda-guidance-documents/requests-feedback-and- meetings-medical-device-submissions-q-submission-program. ↩
-
21 CFR 801 (Labeling) and 21 CFR 809 (In Vitro Diagnostic Products for Human Use). See, e.g., 21 CFR 801.5 (requiring that labeling include adequate directions for use); 21 CFR 801.109(c) (for prescription devices, requiring that labeling include any relevant hazards, contraindications, side effects, and precautions under which practitioners licensed by law to administer the device can use the device safely and for the purpose for which it is intended); and 21 CFR 809.10(b)(6) (for in vitro diagnostic products, requiring labeling accompanying any instruments use or function, installation procedures, performance characteristics and specifications, service and maintenance information, etc.). ↩
-
21 CFR 801.5 (requiring that labeling include adequate directions for use). ↩
-
See sections 515C(a)(3), 515C(b)(3), and 513(f)(2) of the FD&C Act. ↩
-
In accordance with 21 CFR 807.92, “a 510(k) summary shall be in sufficient detail to provide an understanding of the basis for a determination of substantial equivalence.” This includes, but is not limited to, a description of the device, and for those 510(k) submissions in which a determination of substantial equivalence is also based on an assessment of performance data, non-clinical tests, and clinical tests. ↩
-
If a sponsor chooses to submit a 510(k) Statement rather than 510(k) Summary, the sponsor should provide to requestors all PCCP information in the 510(k) that supports transparency to users of FDA’s determination of substantial equivalence for the device and its range of FDA-authorized specifications, as such information constitutes safety and effectiveness information. See 21 CFR 807.93 for requirements on the content and format of a 510(k) Statement. ↩
-
The De Novo decision summary is intended to present an objective and balanced summary of the scientific evidence that served as the basis for the FDA's decision to grant a De Novo request; see https://www.fda.gov/medical-devices/premarket-submissions/de-novo-classification-request. ↩
-
In accordance with 21 CFR 814.9(e), “FDA will make available to the public … a detailed summary of information submitted to FDA respecting the safety and effectiveness of the device that is the subject of the PMA and that is the basis for the order.” ↩
-
Manufacturers are required to comply with the quality system regulation (21 CFR 820). The device and PCCP must be implemented consistent with 21 CFR 820, including, but not limited to: 21 CFR 820.30 Design controls; 21 CFR 820.90 Nonconforming products; and 21 CFR 820.100 Corrective and preventive action. ↩
-
See, e.g., 21 CFR 807.81(a)(3) and 21 CFR 814.39(a). ↩
-
A change to the authorized PCCP could include a change in Description of Modifications, the Modification Protocol, and/or the Impact Assessment, as appropriate. ↩
-
See, e.g., 21 CFR 807.87, 21 CFR 860.220, or 21 CFR 814.20. ↩
-
See sections 513(f)(2) and 515C of the FD&C Act. ↩
-
See FDA’s guidance “Requests for Feedback and Meetings for Medical Device Submissions: The Q-Submission Program” available at https://www.fda.gov/regulatory-information/search-fda-guidance-documents/requests- feedback-and-meetings-medical-device-submissions-q-submission-program.” ↩
-
Section 510(l)(1) and section 515(d)(5)(A)(i) of the FD&C Act. ↩
-
See, e.g., 21 CFR 807.87, 21 CFR 860.220, or 21 CFR 814.20. ↩
-
See sections 513(f)(2) and 515C of the FD&C Act. ↩
-
Section 515C(a)(2) and section 515C(b)(2) FD&C Act. ↩
-
FDA has a long-standing policy of applying the definition of indications for use in the PMA regulation at 21 CFR 814.20(b)(3)(i) in the same way in the 510(k) context. See the FDA guidance “The 510(k) Program: Evaluating Substantial Equivalence in Premarket Notifications [510(k)],” available at https://www.fda.gov/regulatory-information/search-fda-guidance-documents/510k-program-evaluating-substantial-equivalence-premarketnotifications-510k. ↩
-
Section 515C(a)(2)(A) and section 515C(b)(2) FD&C Act. ↩
-
21 CFR Part 820. ↩
-
21 CFR 820 Subpart M – Records. ↩
-
The QS regulation requires manufacturers to retain all records for a period of time equivalent to the design and expected life of the device, but in no case less than 2 years from the date of release for commercial distribution by the manufacturer (21 CFR 820.180(b)). ↩
-
We recommend that manufacturers consider additional characteristics, such as those described in the Blueprint for an AI Bill of Rights (https://www.whitehouse.gov/ostp/ai-bill-of-rights/): race, color, ethnicity, sex (including pregnancy, childbirth, and related medical conditions, gender identity, intersex status, and sexual orientation), religion, age, national origin, disability, veteran status, and genetic information. ↩
-
See 21 CFR 820.180(b). ↩
-
For example, FDA has published a document on “Good Machine Learning Practice for Medical Device Development: Guiding Principles,” available at https://www.fda.gov/medical-devices/software-medical-device- samd/good-machine-learning-practice-medical-device-development-guiding-principles. ↩
-
Section 515C(a)(3) and (b)(3) of the FD&C Act. ↩
-
Section 515C(a)(3) and (b)(3) of the FD&C Act. ↩
-
See 21 CFR 801.5, requiring that labeling include adequate directions for use including statements of all conditions, purposes, or uses for which the device is intended. ↩
-
See section 502(a)(1) of the FD&C Act, stating that a medical device is deemed misbranded if its labeling is false or misleading in any particular. ↩
-
See FDA’s guidance “Multiple Function Device Products: Policy and Considerations” available at https://www.fda.gov/regulatory-information/search-fda-guidance-documents/multiple-function-device-products- policy-and-considerations. ↩
-
Such information would be required in the marketing submission pursuant to 21 CFR 807.81(a)(3) and 21 CFR 814.39(a). ↩
-
For the purposes of this guidance, “part of a hardware device” means the software is used to control a device, or the software is necessary for a hardware device to achieve its intended use. ↩
-
For recommendations related to cybersecurity, please consult FDA guidance documents on this topic, including “Content of Premarket Submissions for Management of Cybersecurity in Medical Devices” (available at https://www.fda.gov/regulatory-information/search-fda-guidance-documents/content-premarket-submissions- management-cybersecurity-medical-devices) and “Postmarket Management of Cybersecurity in Medical Devices” (available at https://www.fda.gov/regulatory-information/search-fda-guidance-documents/postmarket-management- cybersecurity-medical-devices). ↩
-
21 CFR 807.81(a)(3) or 21 CFR 814.39(a). ↩
-
See FDA’s guidance “Requests for Feedback and Meetings for Medical Device Submissions: The Q-Submission Program” available at https://www.fda.gov/regulatory-information/search-fda-guidance-documents/requests- feedback-and-meetings-medical-device-submissions-q-submission-program. ↩
-
The values in this example are shown as variable terms. A completed PCCP should include specific criteria whenever possible. ↩